As you may have seen in the WWDC 2019 videos, Core ML 3 adds a lot of new stuff to machine learning on iOS. The new killer feature is on-device training of models, but it can now also run many advanced model architectures — and thanks to the addition of many new layer types, it should even be able to run new architectures that haven’t been invented yet!
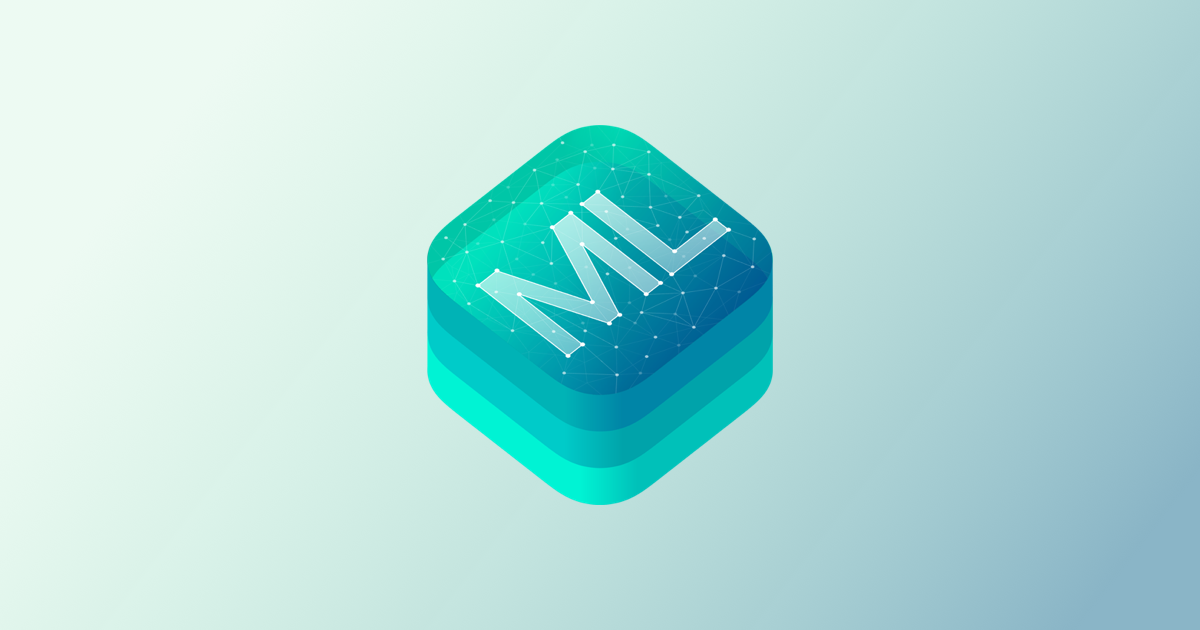
This is by far the biggest update to Core ML since its original release in 2017.
Core ML is not perfect, but with these new additions — as well as the A12 chip’s Neural Engine — Apple is definitely way ahead of everyone else when it comes to machine learning on mobile.
In this blog post I’ll describe in excruciating detail what Core ML 3’s new features are, all the way down to the individual layer types. We’re mostly going to look at the mlmodel format here, not the API from CoreML.framework (which, except for adding training functionality, didn’t really change much).
Do you need to know this stuff if you’re just looking to convert an existing model and use it in your app? Probably not. But it’s definitely good to have as a reference for when you’re designing your own machine learning models that you intend to use with Core ML 3, or when you’re not sure an existing model can be converted.
It’s all in the proto files
So, what is new? If you look at the API documentation for CoreML.framework, you won’t get much wiser. The nitty-gritty isn’t in the API docs but in the Core ML model format specification.
This specification consists of a number of .proto files containing protobuf message definitions. Protobuf, or “protocol buffers”, is the serialization format used by Core ML’s mlmodel files. It’s a common serialization technology, also used by TensorFlow and ONNX. The proto files describe the different objects that can be found in an mlmodel file.
You can find the Core ML model specification here but this website isn’t always up-to-date. It’s better to look directly at the proto files. You can find those inside the coremltools repo. They’re regular text files so you can open them with any editor.
Note: If you’re serious about using Core ML, I suggest getting familiar with the proto files and the protobuf format in general. This is the only place where the capabilities and limitations of Core ML are documented, and you’ll learn a ton by reading through them. You can read more about the mlmodel internals in my book, which I’m updating to include Core ML 3 as we speak.
The main file in the format specification is Model.proto. This defines what a model is, what kind of inputs and outputs a model can have, and what different types of models exist.
An important property of the Model
definition is the specification version. This version number determines which functionality is available in the mlmodel file, and which operating system can run the model.
The new specification version is 4, not 3 as you might expect. There have been three major releases of Core ML, but there was also a small update for iOS 11.2 that bumped the version number.
Core ML models with specification version 4 can only run on iOS 13 and macOS 10.15 (Catalina) or better. If you’re targeting iOS 12 or even 11, forget about using any of the new features shown in this blog post.
Note: When you convert a model, coremltools will choose the lowest possible specification version that your model is compatible with. v3 models can run on iOS 12, v2 models on iOS 11.2, and v1 models on iOS 11.0. Of course, if your model uses any of the newly introduced features, it’s iOS 13 or later only.
New model types
Core ML has always supported the following model types (spec v1):
- Identity — this does nothing, it simply passes the input data through to the output, useful only for testing
- GLM regressor and classifier — for linear and logistic regression
- Support vector machines for regression and classification
- Tree ensemble regressor and classifier — for XGBoost models
- Neural networks — for regression, classification, or general-purpose neural networks
- Pipeline models — these let you combine multiple models into one big model
- Model types for feature engineering — one-hot encoding, imputation of missing values, input vectorization, and so on. These are mostly useful for converting scikit-learn models to Core ML. The model is turned into a Pipeline that has several of these feature engineering models in a row.
Specification version 2 was only a small update that added support for 16-bit floating point weights. Enabling this makes your mlmodel files about 2× smaller but unlike what some people expect, it does not make the model run any faster.
In Core ML 2 (spec v3), the following model types were added:
- Bayesian probit regressor — a more fancy version of logistic regression
- Non-maximum suppression — useful for post-processing object detection results, typically used as the last model in a Pipeline. See my blog post on SSDLite in Core ML for more info.
- VisionFeaturePrint — this is a convolutional neural network for extracting features from images. The output is a 2048-element feature vector. Create ML uses this for transfer learning when training image classifiers, but you can also use it in your own models (such as for image similarity).
- Other models from Create ML: text classifier, word tagger.
- Custom models: sometimes you may have a model type that Core ML doesn’t understand, but you’d still like to put it in a pipeline alongside other models. A custom model lets you put the learned parameters (and any other data) inside the mlmodel file, while you put the actual implementation of the custom logic inside your app.
Other features added by v3 were weight quantization for even smaller mlmodel files (but still no change in inference speed) and flexible input sizes. The API added batch predictions and better support for dealing with sequential data.
OK, that was the story until now. As of Core ML 3 (spec v4), the following model types can also be described by your mlmodel files:
- k-Nearest Neighbors classifier (or k-NN)
- ItemSimilarityRecommender — you can use this to build recommender models, like the one that now comes with Create ML
- SoundAnalysisPreprocessing — this is for Create ML’s new sound classification model. It takes audio samples and converts them to mel spectrograms. This can be used in a Pipeline as the input to an audio feature extraction model (typically a neural network).
- Gazetteer – this is Create ML’s new
MLGazetteer
model, used withNLTagger
from the Natural Language framework. A gazetteer is a fancy look-up table for words and phrases. - WordEmbedding – for Create ML’s new
MLWordEmbedding
model that is a dictionary of words and their embedding vectors; also used with the Natural Language framework. - Linked models — a linked model is simply a reference to another mlmodel file (actually, the compiled version, mlmodelc) in your app bundle. This lets you reuse expensive feature extractors across multiple classifiers — if two different Pipelines use the same linked model, it only gets loaded the once.
The Model
object now has an isUpdatable
property. If this is true
, the model can be trained on-device with new data. This currently only works for neural networks and k-Nearest Neighbors (either as a standalone model or inside a Pipeline).
k-Nearest Neighbors is only a simple algorithm but this makes it quite suitable for on-device training. A common method is to have a fixed neural network, such as VisionFeaturePrint, extract the features from the input data, and then use k-NN to classify those feature vectors. Such a model is really fast to “train” because k-NN simply memorizes any examples you give it — it doesn’t do any actual learning.
One downside of k-NN is that making predictions becomes slow when you have a lot of examples memorized, but Core ML supports a K-D tree variant that should be quite efficient.
What’s new in neural networks
This is where it gets interesting… the vast majority of changes in Core ML 3 are related to neural networks.
Where Core ML 2 supported “only” about 40 different layer types, Core ML 3 adds over 100 new ones. But let’s not get carried away, some of these new layers are merely refinements of older layer types to make them suitable for handling flexible tensor shapes.
For Core ML 2 and earlier, the data that flowed through the neural network always was a tensor of rank 5. That means each tensor was made up of the following five dimensions, in this order:
(sequence length, batch size, channels, height, width)
This choice makes a lot of sense when the input to your neural network is mostly images, but it’s not very accommodating to other types of data.
For example, in a neural network that processes 1-dimensional vectors, you were supposed to use the “channels” dimension to describe the size of the vector and set the other dimensions to size 1. In that case, the shape of the input tensor would be (1, batch size, number of elements, 1, 1)
. That’s just awkward.
Many of the new layers that were added in Core ML 3 support tensors of arbitrary rank and shape, making Core ML much more suitable to data other than images.
Tip: This is why it’s important to know how to read the proto files, as they explain for each layer how tensors of different ranks are handled. This kind of thing isn’t documented anywhere else!
All the neural network stuff is described in NeuralNetwork.proto. It’s a big file at almost 5000 lines…
The main object is NeuralNetwork
, although there are two other variations: NeuralNetworkClassifier
and NeuralNetworkRegressor
. The difference is that a plain neural network outputs MultiArray objects or images, while the classifier variant outputs a dictionary with the classes and their predicted probabilities, and the regressor simply outputs a numeric value. Other than that small difference in how the output is interpreted, these three model types all work the same.
The NeuralNetwork
object has a list of layers, as well as a list of preprocessing options for any image inputs. Core ML 3 adds a few new properties that describe:
- how inputs of type MultiArray are converted into tensors. You can choose between the old way, which creates that rank-5 tensor shown previously, or the new way, which simply passes the input tensor through unchanged. For most types of data that is not images, it makes sense to use this new method.
- how image inputs are converted into tensors. Instead of the old rank-5 tensor, you can choose to use a rank-4 tensor, which is just
(batch size, channels, height, width)
. This drops the “sequence length” dimension, which you usually don’t need for images (unless, of course, you have a sequence of them). - the hyperparameters for training this model, if you chose to enable that. This is a
NetworkUpdateParameters
object (described below).
I plan to write a detailed blog post about on-device training soon, but just to give you an idea of what is involved:
- The
isUpdatable
property ofModel
must be set to true. - The
isUpdatable
property of any layers that you wish to train must be set to true. This allows you to limit training to specific layers only. Currently, training is only supported for convolution and fully-connected layers. - The
WeightParams
objects that hold the learnable parameters for the layers you wish to train must also have theirisUpdatable
property set. - You need to define additional “training inputs” on the model that are used to provide the ground-truth labels to the loss function.
In addition, the NetworkUpdateParameters
object describes:
- which loss function(s) to use — supported loss functions are categorical cross entropy and MSE. Inside the mlmodel file, a loss function is just another layer. It only has two properties: the name of one of the model’s output layers, and the name of the model’s training input that provides the target labels. For cross entropy loss, the input must be connected to the output of a softmax layer.
- what optimizer to use — currently only SGD and Adam are supported
- the number of epochs to train for
Update: New in beta 3 are shuffle
and seed
parameters that tell Core ML to randomly shuffle the training data before each epoch.
Note that hyperparameters such as the number of epochs, the learning rate, and so on, can be overridden inside the app. The values inside the mlmodel should be set to reasonable defaults but you’re not stuck with them if you don’t like them.
Neural network layers in Core ML 2
Now let’s get to the good stuff: the neural network layers. The first versions of Core ML supported only the following layer types:
- Convolution: 2D only, although you can fake 1D convolution by setting the kernel width or height to 1. Also supports dilated or atrous convolutions, grouped (depthwise) convolution, and deconvolution.
- Pooling: max, average, L2, and global pooling.
- Fully-connected, also known as “inner product” or “dense” layer.
- Activation functions: linear, ReLU, leaky ReLU, thresholded ReLU, PReLU, tanh, scaled tanh, sigmoid, hard sigmoid, ELU, softsign, softplus, parametric soft plus. All the different activation functions are handled by a single layer type,
ActivationParams
. Note that, unlike in Keras, where the activation can be a property of the convolution layer, in Core ML they are always layers of their own. For extra speed, the Core ML runtime will “fuse” the activation function with the preceding layer, if possible. - Batch normalization.
- Other types of normalization, such as using mean & variance, L2 norm, and local response normalization (LRN).
- Softmax: usually the last layer of a
NeuralNetworkClassifier
object. - Padding: for adding extra zero-padding around the edges of the image tensor. Convolution and pooling layers can already take care of padding themselves, but with this layer you can do things such as reflection or replication padding.
- Cropping: for removing pixels around the edges of the tensor.
- Upsampling: nearest neighbor or bilinear upsampling by an integer scaling factor.
- Unary operations: sqrt, 1/sqrt, 1/x, x^power, exp, log, abs, thresholding.
- Element-wise operations between two or more tensors: add, multiply, average, maximum, minimum. These support broadcasting to some extent.
- Element-wise operations on a single tensor: multiply by a scale factor, add bias. These support broadcasting.
- Reduction operations on a single tensor: sum, sum of natural logarithm, sum of squares, average, product, L1 norm, L2 norm, maximum, minimum, argmax.
- Dot product between two vectors, can also compute cosine similarity.
- Layers that reorganize the contents of a tensor: reshape, flatten, permute, space-to-depth, depth-to-space.
- Concat, split, and slice: these combine or pull apart tensors.
- Recurrent neural network layers: basic RNN, uni- and bi-directional LSTM, GRU (unidirectional only).
- Sequence repeat: duplicates the given input sequence a number of times.
- Embeddings.
- Load constant: can be used to provide data to some of the other layers, for example anchor boxes in an object detection model.
Specification version 2 added support for custom layers in neural networks. This was a very welcome addition, as now it became possible to convert many more models.
Inside the mlmodel file, a custom layer is simply a placeholder, possibly with trained weights and configuration parameters. In your app, you’re supposed to provide a Swift or Objective-C implementation of the layer’s functionality, and possibly a Metal version as well for running it on the GPU. (Unfortunately, the Neural Engine isn’t currently an option for custom layers.)
For example, if a model requires an activation function that is not in the list above, it can be implemented as a custom layer. However, you can also do this by cleverly combining some of the other layer types. For example, a ReLU6 can be made by first doing a regular ReLU, then multiplying the data by -1, thresholding to -6, and finally multiplying by -1 again. That requires 4 different layers but — in theory — the Core ML framework could optimize this away at runtime.
In Core ML 2 (spec v3), the following layer types were added:
- Resize bilinear: unlike the upsampling layer, which only accepts integer scaling factors, this lets you perform a bilinear resize to an arbitrary image size.
- Crop-resize: for extracting regions of interest from a tensor. This can be used to implement an RoI Align layer as used in Mask R-CNN.
As you can tell, even though there are some layer types for dealing with vectors or sequence data, most of the layers are very focused on convolutional neural networks for dealing with images. In Core ML 2, all the layers expect tensors of shape (sequence length, batch size, channels, height, width)
even if your data is only one-dimensional.
Core ML 3 (spec v4) relaxes that requirement a little bit for these existing layer types. For example, an inner product layer can now work on input tensors from rank 1 to rank 5. So in addition to adding a whole bunch of new layers, Core ML 3 also made the existing layer types more flexible.
Note: The doc comments inside NeuralNetwork.proto explain for each of these layers how tensors of different ranks are handled. If you’re ever wondering what the right tensor shape is for a layer, that’s the place to look.
Now let’s finally look at the new stuff!
The new neural network layers
Over 100 new layers… phew! It’s a lot but I’m going to list them all because it is useful to have this blog post as a reference. (Of course, the proto files are the authoritative source.)
Keep in mind that in the previous version of the spec, certain operations were combined into a single layer. For example, all the unary tensor operations were part of the layer type UnaryFunctionLayer
. But with Core ML 3, a whole bunch of new unary operations were added and they all have their own layer type, which obviously inflates the total count.
Note: In the proto files, the name of every layer type ends with Params
, so the unary function layer type is really named UnaryFunctionLayerParams
. For the sake of readability, I’m dropping the “params” from the layer names here.
Core ML 3 adds the following layers for element-wise unary operations:
ClipLayer
: clamps the input between a minimum and maximum valueCeilLayer
andFloorLayer
: the usual ceil and floor functions applied to an entire tensor at onceSignLayer
: tells you whether a number is positive, zero, or negativeRoundLayer
: rounds off the values of a tensor to whole numbersExp2Layer
: calculates2^x
for every element in the tensorSinLayer
,CosLayer
,TanLayer
,AsinLayer
,AcosLayer
,AtanLayer
,SinhLayer
,CoshLayer
,TanhLayer
,AsinhLayer
,AcoshLayer
,AtanhLayer
: the well-known (hyperbolic) trig functionsErfLayer
: computes the Gauss error function
This seriously expands the number of math primitives supported by Core ML. Unlike the math functions it already had, these can deal with tensors of any rank.
There is only one new activation function:
GeluLayer
: the Gaussian error linear unit activation, either exact or using a tanh or sigmoid approximation
Of course, you can use any unary function as an activation function, or create one by combining different math layers.
There are also new layer types for comparing tensors:
EqualLayer
,NotEqualLayer
,LessThanLayer
,LessEqualLayer
,GreaterThanLayer
,GreaterEqualLayer
LogicalOrLayer
,LogicalXorLayer
,LogicalNotLayer
,LogicalAndLayer
These output a new tensor that is 1.0
where the condition is true, and 0.0
where the condition is false (also known as a tensor “mask”). These layer types support broadcasting, so you can compare tensors of different ranks. You can also compare a tensor with a (hardcoded) scalar value.
One place where these layer types come in useful is with the new control flow operations (see below), so that you can branch based on the outcome of a comparison, or create a loop that keeps repeating until a certain condition becomes false.
Previously, there were a handful of layers for element-wise operations between two or more tensors. Core ML 3 adds a few new types, and — as you can tell from the name — these are now much more flexible because they fully support NumPy-style broadcasting:
AddBroadcastableLayer
: additionSubtractBroadcastableLayer
: subtractionMultiplyBroadcastableLayer
: multiplicationDivideBroadcastableLayer
: divisionFloorDivBroadcastableLayer
: division followed by rounding down, to get a whole number resultModBroadcastableLayer
: remainder of divisionPowBroadcastableLayer
: raise the first tensor to the power of the secondMinBroadcastableLayer
,MaxBroadcastableLayer
: minimum and maximum
Reduction operations have now moved into their own layers. Core ML already supported most or all of these, but these new versions can work with arbitrary tensors, not just images. You can now do the reduction over one or more axes.
ReduceSumLayer
: compute the sum over the specified axesReduceSumSquareLayer
: compute the sum of the squares of the tensor’s elementsReduceLogSumLayer
: compute the sum of the natural logarithm of the elementsReduceLogSumExpLayer
: the log-sum-exp trick! Exponentiate the elements, sum them up, and take the natural logarithm.ReduceMeanLayer
: compute the average of the elementsReduceProdLayer
: multiply all the elements togetherReduceL1Layer
,ReduceL2Layer
: compute the L1 or L2 normReduceMaxLayer
,ReduceMinLayer
: find the maximum or minimum valueArgMaxLayer
,ArgMinLayer
: find the index of the maximum or minimum valueTopKLayer
: find the k top (or bottom) values and their indices; this is a more general version of argmax and argmin. The value of k can be provided by an input, so it does not have to be hardcoded into the model.
Speaking of math stuff, Core ML 3 also adds the following:
BatchedMatMulLayer
: a general-purpose matrix multiplication on two input tensors, or between a single input tensor and a fixed set of weights (plus an optional bias). Supports broadcasting and can transpose the inputs before doing the multiplication. In other words, gemm.LayerNormalizationLayerParams
: a simple normalization layer that subtracts beta (such as the mean) and divides by gamma (e.g. the standard deviation), both of which are provided as fixed weights. This is different from the existingMeanVarianceNormalizeLayer
, which performs the same formula but actually computes the mean and variance from the tensor at inference time.
A number of other existing operations have been extended to use arbitrary size tensors, also known as rank-N tensors or N-dimensional tensors. You can recognize such layer types by the “ND
” in their name:
SoftmaxNDLayer
: the old softmax could only be applied to the channel axis, this one can use any axisConcatNDLayer
: concatenate two or more outputs across any axisSplitNDLayer
: the opposite of concat. Previously you could only split on the channel axis, and only into parts with the same size. Now it lets you split on any axis and the sizes of the splits can be different.TransposeLayerParams
: OK, this doesn’t have ND in its name but it’s the same asPermuteLayer
except it supports N-dimensional tensorsEmbeddingNDLayer
: like the existingEmbeddingLayer
but with more flexible tensor shapesLoadConstantNDLayerParams
: like the existingLoadConstant
layer but with more flexible tensor shapes
Slicing lets you keep only a part of the original tensor and throw away the rest. The old slicing layer could slice the input tensor across the width, height, or channel axis. Core ML 3 gives us two new slicing layers that support slicing across any axis:
SliceStaticLayer
SliceDynamicLayer
I’m not 100% sure yet how these layers work as the documentation isn’t very helpful, but it looks like they can slice by indices or by a mask. In any case, these layers slice and dice!
Why two different versions? You’ll actually see this distinction between static and dynamic in some of the upcoming layer types too.
Static basically means, “everything is known about this operation beforehand” while dynamic means “the arguments of this operation can change between runs”. For example, the static version of a layer may have a hardcoded outputShape
property while the dynamic version can use a different output shape every time.
Note: In the first version of Core ML, the size of the input was hardcoded — for example, only 224×224 images. Since version 2, Core ML has supported flexible input shapes, where you can tell the mlmodel that a given input can accept tensors between a minimum and maximum size, or from a list of predefined sizes. That sounds pretty dynamic! However, by dynamic operations in Core ML 3 we mean something slightly different…
Here “dynamic” means that inside the graph itself, from one run to the next, the shapes of the intermediate tensors may be different, even if the input tensor is always of the same size.
For example, if the first part of your model predicts a bounding box that then gets cropped and fed to the second part of your model, it’s likely that this bounding box and the resulting cropped tensor will have a different size every time. Therefore, the layers in the second part of the model cannot make any assumptions about the shape of that cropped tensor.
Because Core ML is no longer limited to static image-based models but now also contains methods for control flow and other dynamic operations, it has to be able to manipulate tensors in all kinds of fancy ways. Let’s look at those functions.
GetShapeLayer
: this returns a vector containing the shape of the input tensor, which lets you inspect at runtime how big a given tensor isBroadcastToStaticLayer
,BroadcastToLikeLayer
,BroadcastToDynamicLayer
: these change the shape of the tensor according to the common NumPy broadcasting rulesRangeStaticLayer
,RangeDynamicLayer
: fills the tensor with evenly spaced values in a given interval, much like NumPy’sarange()
function.FillStaticLayer
,FillLikeLayer
,FillDynamicLayer
: these functions fill the tensor with a constant scalar value — usually all zeros or ones, but any floating point value will do.
Notice how some of these layer types come in three different variants: Like
, Static
, and Dynamic
. What do these mean?
Static is the simplest one: all the properties for this layer are hardcoded in the mlmodel file. If you know that, regardless of what happens, you’re always going to need a tensor with shape
(32, 10, 7)
, you would useFillStaticLayer
. Note thatFillStaticLayer
andRangeStaticLayer
do not take an input tensor, butBroadcastToStaticLayer
does.Like takes an additional input tensor and outputs new a tensor that has the same shape as that input. The layer ignores the actual values from that extra input tensor — it only looks at its shape.
FillLikeLayer
andRangeLikeLayer
take only one input and use this to determine the shape of the output tensor, whileBroadcastToLikeLayer
takes two input tensors: the one to broadcast, and the second one whose shape it will broadcast to.Dynamic is similar to like: it also takes an additional input tensor, but this time it’s not the shape of that tensor that’s important but its contents. For example, to fill a tensor of shape
(32, 10, 7)
you would pass in a tensor of shape(3)
that has three values:32
,10
, and7
. Interestingly,FillDynamicLayer
doesn’t let you pass in the scalar value dynamically.
Note: Static / dynamic isn’t always about the output shape, it depends on the layer. For example, in the random distribution layers (see next), you can set the random seed dynamically too. Some of the dynamic layers have several different inputs that let you override their default properties.
Core ML 3 also lets you create new tensors by sampling from random distributions:
RandomNormalStaticLayer
,...LikeLayer
,...DynamicLayer
RandomUniformStaticLayer
,...LikeLayer
,...DynamicLayer
RandomBernoulliStaticLayer
,...LikeLayer
,...DynamicLayer
CategoricalDistributionLayer
There were already layers for reshaping and flattening layers, but more variants have been added:
SqueezeLayer
: remove any dimensions that have size 1ExpandDimsLayer
: the opposite of squeeze, adds new dimensions with size 1FlattenTo2DLayer
: flatten the input tensor into a two-dimensional matrixReshapeStaticLayer
,ReshapeLikeLayer
,ReshapeDynamicLayer
RankPreservingReshapeLayer
: this is like usingreshape(..., -1)
in NumPy. The layer automatically infers the rest of the new shape. Handy!
Besides concat and split operations for arbitrary tensors, Core ML 3 also adds the following tensor manipulation operations:
TileLayer
: repeat the tensor a given number of timesStackLayer
: join tensors along a new axis (as opposed to concat, which joins the tensors along an existing axis)ReverseLayer
: reverses one or more dimensions of the input tensorReverseSeqLayer
: reverses the sequence, for tensors that store a sequence of dataSlidingWindowsLayer
: slides a window over the input data and returns a new tensor with the contents of the window at every step
Also new is support for gather and scatter:
GatherLayer
,GatherNDLayer
,GatherAlongAxisLayer
: given a set of indices, keeps only the parts of the input tensor at those indicesScatterLayer
,ScatterNDLayer
,ScatterAlongAxisLayer
: copies the values of one tensor into another tensor, but only at the given indices. Besides copying there are also other accumulation modes: add, subtract, multiply, divide, maximum, and minimum.
Speaking of selecting elements based on some condition, here are a few more layer types for dealing with masks:
WhereNonZeroLayer
: creates a new tensor with only the elements that were not zero. You could use this with a mask tensor from a tensor comparison, such asLessThanLayer
, for example.WhereBroadcastableLayer
: takes three input tensors, two data tensors and a mask that contains ones (true) or zeros (false). Returns a new tensor containing the elements of the first data tensor or the second data tensor, depending on whether the value from the mask is true or false.UpperTriangularLayer
,LowerTriangularLayer
: zeroes out the elements below or above the diagonalMatrixBandPartLayer
: zeroes out the elements outside a central band
Beta 3 of coremltools 3.0 snuck in a few new layer types:
ConstantPaddingLayer
: adds a certain amount of padding around a tensor. Unlike the existing padding layer, this one works for any axis, not just the width and height dimensions.NonMaximumSuppressionLayer
: there already was a separate model type for doing NMS on bounding boxes, which you’d put into a pipeline following an object detect detection model, but now it’s also possible to do NMS directly inside the neural network.
Finally — and perhaps most excitingly — Core ML 3 adds layers for control flow such as decision making and loops.
Previously, Core ML would run the neural network graph from top to bottom just once for each prediction. But now it can repeat certain parts of the graph and skip others. Exactly which parts of the neural network get executed by Core ML can vary between one run of the model and the next — this depends purely on the contents of your input data.
The control flow layers are:
BranchLayer
: this is like an if-else statement. It contains twoNeuralNetwork
objects, one that runs when the input to this layer is true, the other when the input is false. Yep, you read that correctly: a branch contains a smaller neural network inside the main neural network. Because Core ML doesn’t have a boolean tensor type, you’ll actually pass in 1 or 0 instead of true or false. (Core ML considers the condition to the true if the value is greater than 1e-6.)LoopLayer
: this is like a while loop. If no input is given, the loop repeats for the maximum number of iterations specified in the layer. You can override this by passing in the number of iterations you want to loop for. TheLoopLayer
contains a “body”NeuralNetwork
that represents the inside of the while loop. The layers from this neural net are run on every iteration. It’s also possible to include aNeuralNetwork
that acts as the condition of the while loop. This “condition” neural network is run once before the loop starts and again before every new iteration. As long as it outputs a value greater than 0, the loop keeps repeating.LoopBreakLayer
: you can put this into the loop’s bodyNeuralNetwork
to terminate the loop, just like a regular break statement.LoopContinueLayer
: you’d put this into the bodyNeuralNetwork
if you want to stop the current loop iteration and skip ahead to the next one, just like a regular continue statement.CopyLayer
: this is used to overwrite a previous tensor, for example to replace an old result with a new one — without thisCopyLayer
, tensors in the graph could never change once they have been computed.
Note that the BranchLayer
and LoopLayer
do not have outputs. They always pass control to one of their child NeuralNetwork
objects, which will have an output of some kind. (I haven’t tried it, but it seems reasonable to assume you can nest these loops and branches too.)
For an example of how to use these new control flow layers, check out this Jupyter notebook from the coremltools repo. It shows how to implement a simple iterative process inside the Core ML model and uses a many of the new layer types.
The example works like this:
- use a
LoadConstantND
layer to load the value0
into the output namediteration_count
- add a
LoopLayer
that will loop for a certain maximum number of iterations - inside the loop, add a new neural network that performs some kind of computation
- at the end of the computation, use a arithmetic layer to increment the current value from
iteration_count
, and then aCopyLayer
to overwrite the value inside theiteration_count
output - they also use another
CopyLayer
to copy the result of the computation back into the original tensor, so that the next iteration of the loop can use this new value - add a
LessThanLayer
to compare the output of the computation to some convergence threshold, and feed this yes/no result into aBranchLayer
- add a new neural network to the
BranchLayer
that just hasLoopBreakLayer
inside it. In other words, if the branch happens — because the output of the computation was small enough to go under the convergence threshold — then we’ll break out of the loop.
It’s a little weird perhaps, but very flexible! Key point is to remember to use the CopyLayer
to overwrite existing tensors with new values, much like an assignment statement in Swift. After you run the model, the iteration_count
output will now have counted how many times the loop was repeated. Of course, this count may be different every time, depending on the values of the inputs to the model, as some will converge quicker than others. Pretty cool!
Thanks to these control flow layers, Core ML 3 graphs can go way beyond the traditional acyclic graphs. However, you only get branches and loops — there is currently no such thing as a “goto”. Core ML is not Turing-complete quite yet. 😁
At the very bottom of NeuralNetwork.proto are the layer definitions for on-device training. We already briefly looked at those, but here they are again:
LossLayer
,CategoricalCrossEntropyLossLayer
,MeanSquaredErrorLossLayer
: the loss functionsOptimizer
,SGDOptimizer
,AdamOptimizer
: the available optimizers
Note: I find it a little odd that the loss function is defined in the mlmodel file. This makes it impossible to train with other loss functions. Likewise for the optimizers. Perhaps a future version of Core ML will allow us to provide custom implementations of these.
And that’s it, those are all the new layer types in Core ML 3!
Most of the new layer types are for creating, shaping, and manipulating tensors. There are also many new mathematics primitives. Not a whole lot of “real” neural network stuff has been added. But having these low-level operations will make it a lot easier to support all kinds of new, still unimagined, layer types.
Then again, if implementing a new layer type requires adding 20 different math layers to your Core ML mlmodel, it might be faster to write a custom layer… 😉
First published on Saturday, 8 June 2019.
If you liked this post, say hi on Twitter @mhollemans or LinkedIn.
Find the source code on my GitHub.
New e-book: Code Your Own Synth Plug-Ins With C++ and JUCE
Interested in how computers make sound? Learn the fundamentals of audio programming by building a fully-featured software synthesizer plug-in, with every step explained in detail. Not too much math, lots of in-depth information! Get the book at Leanpub.com